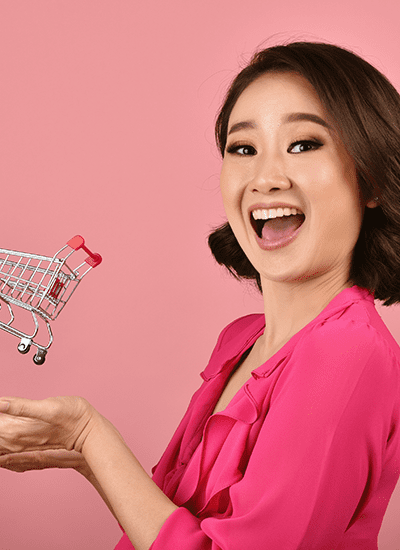
What Are the Benefits of Advanced Machine Learning for 1-To-1 Personalization?
In a previous blog, we explored why advanced machine learning matters for personalization overall. But, how does advanced machine learning impact 1-to-1 personalization?
Because Machine Learning has become a widely used and highly evolved technology, advanced Machine Learning has become increasingly important to personalization software. In this post, we’ll take a deeper look at why.
Advanced Machine Learning – a Definition
All Machine Learning (ML) takes inputs from its environment and uses them to improve its performance when executing a given task. But classical ML is a lot more labor-intensive than advanced ML. This is because it needs human teams to oversee the input of data and guide the learning algorithm when making decisions.
Advanced ML generally breaks down into two categories: deep learning and reinforcement learning. Whereas deep learning layers neural networks to filter results, progressively becoming more and more accurate, reinforcement learning uses one neural network layer, which uses inputs to make decisions, and then learns from the results of those decisions to arrive at progressively better decisions.
It learns by a system of rewards and punishments, with more correct decisions being rewarded and fewer correct ones being punished.
What are the benefits of advanced ML to personalization?
It’s this interaction with feedback from the real world that sets advanced ML apart from other types of ML and makes it the ideal type of ML for personalization in eCommerce.
Advanced ML has several benefits for eCommerce marketers:
- Create 1-to-1 Personalization at Scale – This is where advanced ML makes the biggest impact. The huge data sets available through first and third-party data enable ML to make incredibly accurate decisions about how to personalize at the level of the individual customer. It’s impossible for teams of marketers to make these granular decisions when working at scale. Advanced ML is what makes 1-to-1 personalization possible.
- Easy to Use – Because there’s less oversight required, advanced ML is much more straightforward to set up and deploy. You set up an advanced personalization ML very quickly with behavioral targets that have been pre-defined and then, when you’re ready, feed in your own customer data to deliver a richer personalized experience to your visitors.
- Easy to Understand – With a reinforcement learning model, marketers can see the decisioning process in action. This is different from deep learning, which is often described as a ‘black box.’ As reinforcement learning takes an iterative approach, you can see how decisions are made every step of the way and on what basis those decisions are being improved on.
- Flexible – For the same reason, it’s easy for marketing and business teams to step in and make decisions themselves as the need arises. The combination of personalization choices based on the results of ML decisions and human teams produces powerful 1-to-1 experiences for customers.
- Multiple Use Cases – Some ML models are rigid in what they can be applied to. With advanced reinforcement learning models, the learning from one area can be carried over to others. In practice, it means that ML isn’t just for product recommendations, you can use advanced ML at all stages of the customer journey, from search to social proof. You can also use ML to carry out decisions on multiple channels, ensuring consistency across touchpoints.
User Stories
Now that we’ve got an understanding of what advanced ML is and how it can benefit marketers seeking 1-to-1 personalization, let’s look at some of the brands that have successfully integrated advanced ML into their customer journeys.
Reebok
As one of the world’s most recognizable brands, Reebok has millions of customers across the globe. These customers are incredibly diverse: they are geographically dispersed, come from different social backgrounds, are of various ages, and have different holiday seasons. Reebok, therefore, needed to find a way to cater to their varied customer base, giving them an individual, unique experience when they visited their site. They turned to Monetate Personalization, which has advanced ML at its heart, to help them.
They wanted to:
- Use ML to deliver personalization at scale by predicting user intent
- Create a consistent, relevant customer journey across touchpoints
- Allow ML decisions based on customer data from a variety of sources
The main focus for realizing these ambitions was their product description pages. Their ML-powered personalization software redesigned their product pages, changing the layout, order, and messaging depending on who was visiting them.
As a result, they saw a 4.2% increase in revenue per session on Black Friday 2021 compared to 2020, a 5.5% increase in add-to-cart rate, and a 12.2% increase in click-through rate.
Helly Hansen
Similar to Reebok, Helly Hansen has a very varied customer base, split across continents and demographics. They too needed a personalization solution that would create unique and relevant experiences for their customers, regardless of where in the world they were visiting their site from or what time of year it was.
They partnered with Monetate and utilized slotted recommendations. This allowed them to have four product recommendation slots on their homepage, each of which displays a product based on automated personalization and dynamic testing.
The results: they enjoyed a 28% increase in revenue per session after implementing slotted recommendations, decreased returning customer bounce rates by 4% with 1-to-1 personalization on their homepage, and achieved 50% more clicks than previously when they switched to Monetate Personalization.
Room & Board
Room & Board has 17 different customer segments, which meant their personalization process was a laborious one. With the help of Monetate’s ML-powered decisioning engine, they were able to scale their personalization across customer segments.
They used the advanced ML technology to decide which hero image should be displayed on their homepage, leveraging their large pool of customer data and 40+ behavioral and contextual targets.
This hyper-relevant content, delivered in real-time, produced an 80% lift in their conversions, a 50% increase in homepage engagement, and a 10% boost in average order value for visitors engaging in personalized content.
Find out what advanced ML can bring to your personalization strategy
If these customer stories have whetted your appetite and piqued your curiosity, you can find out more about the technology powering advanced ML, what it means for 1-to-1 personalization, and what it can bring to your brand in our downloadable eBook.
If you’re interested in learning more about 1-to-1 personalization with Monetate Personalization, we encourage you to request a demo.