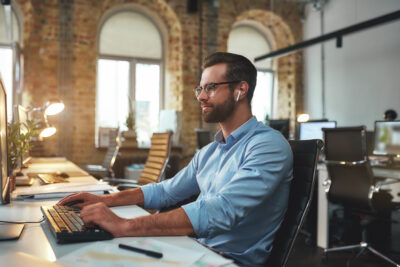
SEO A/B Split Testing Guide: How to Do It Properly
While the science behind search engine visibility has never been murkier, website owners have a new tool that shines light into the darkness: SEO A/B testing. While SEO testing requires resources, expertise, and plenty of website traffic to implement, it provides an accurate way to measure the impact of content and SEO investments.
Search results are more personalized than ever before, with variations in organic page-one results determined by a host of factors, including device, location, prior browsing history, and more. In this environment, it’s hard to know whether or when your website “ranks well.” By capturing and comparing inbound traffic in a controlled experiment, SEO A/B testing takes the guesswork out of your efforts.
Does A/B Testing Negatively Affect SEO?
Because SEO success depends on so many factors, it can be tempting simply to make changes and measure the impact with a simple comparison over time. But this “before and after testing” has serious limitations.
For one, changes in SEO performance between two time periods may be the result of seasonality rather than site optimization. If the “before” period is farther from the end-of-year peak season for a retailer, for example, then the “after” timeframe may show better results simply because more buyers are starting to shop for holiday gifts. The timing of other marketing activities – such as new catalog drops, popular email campaigns, or press coverage – can likewise impact “before” vs “after” performance, as can external factors such as a change to search engine algorithms, instability in the economy, or even a natural disaster.
A/B testing eliminates the inconsistency of comparing performance over separate timeframes. Instead, new changes are tested alongside the originals at the same time, creating a controlled environment for measuring performance impact. Standard A/B tests accomplish this simultaneous comparison by serving each version to a random subset of the website audience. For example, a retailer who wants to gauge the impact of a change to the “buy” button on a product page would create two versions of the same page and split incoming traffic between them.
When it comes to measuring SEO performance, however, the audience isn’t people, but a handful of search engine crawlers – and with Google commanding more than 90% of the search market, the GoogleBot is the algorithm that matters most. Since this audience of one can’t be split, A/B testing takes another form.
Rather than show two versions of the same page, SEO split testing compares test and original groups of pages with the same function – such as all product pages within a particular category on an eCommerce site. Changes are implemented on all the pages in the test group, while the original, or control, group is left as-is. SEO performance is then monitored for each group during the limited test period.
This method avoids negative A/B testing impact on SEO, as outlined in Google’s pointers:
- Cloaking is when website code directs search engine crawlers to interact with a different set of content than what human viewers actually see. The practice runs afoul of Google’s guidelines and can result in demotion or removal from search results.
- Duplicate content occurs when the same material is posted on multiple pages within a site, confusing search engine crawlers. Using the “canonical” tag to indicate the definitive page version helps flag which content the bots should crawl.
Prerequisites for SEO Experiments
SEO split testing can produce valuable insights – if companies meet specific criteria. Without them, testing may be inconclusive or too resource-intensive to boost the bottom line. Consider these prerequisites:
- Website traffic: SEO split testing is intended for larger sites with hundreds of thousands of sessions per month. With more traffic, you need less time to produce statistically significant results, and any temporary swings in user behavior are absorbed as outliers. If your traffic patterns are stable, you may be able to make do with less traffic, but if you experience seasonal or even weekly variability, bigger is better.
- Templatized website pages: Your website needs enough pages that are similar to create a control and a test group, and those pages collectively need to generate significant traffic – at least 30,000 sessions per month, accordingly to SearchPilot. Ecommerce, travel, and media sites with hundreds of template-based pages are ideal.
- Mature SEO capabilities: To make the most of SEO split testing, your marketing team should be adept at web analytics, interpreting data, and SEO optimization techniques. These skills are necessary to derive actionable strategies from test results and realize benefits to the bottom line.
If your business doesn’t meet the criteria, consider focusing first on basic SEO best practices, and reconsidering testing once you’ve grown your audience and run out of proven techniques to implement.
How to Create an Effective SEO A/B Test
Assuming you’re in a position to make the most of SEO A/B testing, the first step is to design an effective test. To generate clear and measurable results, follow these steps:
1. Start with a baseline.
Use existing reporting to create a snapshot of current performance and to create a realistic goal for improvement.
2. Choose what to test.
As you learn the ropes of SEO split testing, start with simpler experiments, and then work toward more complicated trials as your expertise grows. For example, adjustments to verbiage in the <TITLE> or <META> tags or headlines are among the most straightforward elements to test, along with adjustments to the position of existing content.
A new page content structure or additional page markup, such as tagging a FAQ, is more complex to test; the changes are widespread, making it hard to pinpoint which exact element impacts performance. Still more tricky is tracking the cascading sitewide effects of a change such as altering or adding internal links or redesigning the product recommendations carousel on an eCommerce site.
3. Formulate a hypothesis.
A solid hypothesis lays the groundwork for testing success. You gain clarity and accountability when you write out what you already know, specify which metrics you believe the change will impact, and describe the test’s design and duration.
Use your hypothesis to check that your focus is tight enough. Ideally, each test should track the impact of a single change so that the results send a clear signal. Additionally, spelling out how you’ll measure results helps you build the test to collect the right data. You can also check your hypothesis for faults to ensure you avoid the pitfalls that cause A/B test failure.
Properly Execute SEO Testing
Setting up an SEO split test is more complicated than a standard A/B test because you need more than a single page and its variation. While it’s theoretically possible to manage manually the page groupings and the archive of original and test page versions, dynamic content management and testing systems can ease administration and avoid extra resource costs. With the sunsetting of Google Optimize, practitioners must now integrate third-party testing and tracking tools to conduct A/B testing in Google Analytics 4. To set up the test:
1. Select control and variant pages.
To sort the relevant set of content into test and control groups, start with the pages within the category that meet the threshold for adequate traffic, then strive to make both groups statistically similar.
A common misconception is that you should assign pages to groups randomly, but if you do, you risk skewing results if popular pages all happen to land in one group. Instead, use your website analytics tool to carefully search, sort, and assign pages to groups.
In addition to traffic, consider metrics such as orders and time on site. If you’re testing eCommerce site product pages, for example, you should ensure there are equal numbers of best sellers in each group. Strive for an equal balance of seasonal or timely items in each group as well; the mix of test pages for an apparel site shouldn’t include all shorts in one group and all winter outerwear in another, but an assortment of both product types in each group.
You can manually sort pages into groups based on your website analytics, or consider specialized testing tools that automate the job. Monetate’s A/B testing and experimentation solution uses artificial intelligence (AI) to process behavioral data and suggest groupings, as well as enabling custom group creation for any part of the website experience.
2. Enact changes on the variant group of pages.
Roll out the change whose impact you want to measure across the subset of pages you’ve designated as variables. You can either make these changes manually or use settings within your content management system to create a subcategory with page templates of its own to alter.
Just remember that if the test finds no improvement or – worse – a performance decline for pages in the variant group, you need a means to roll back the changes. Keep track of which pages you change and make backup copies of the originals. Or use your content management system to move pages back into the main category and reassign them to the previously existing template.
3. Set a realistic timeframe.
Because the aim of SEO split testing is to measure impact on search visibility, you need to run the test for as long as it takes search engine crawlers to index all the pages in the test group and for those changes to generate enough traffic that any impact is statistically significant. Accordingly to SEOTesting, simpler tests such as the <TITLE> tag changes mentioned above can show valid results in two weeks, while the most complex tests dealing with changes to internal linking hierarchies may take two months to fully reflect changes. The median of four to six weeks is generally considered safe.
Technical Aspects of Organic Split Testing
As you set up your SEO split testing, consider how the back-end technology will work to serve test versions of pages, and which method works best for your website platform and testing tools.
- With server-side testing, your website host server directly launches a test or a control version of the page based upon the user’s browser request – or, in the case of SEO testing, the search engine crawler request.
- Client-side testing, by contrast, uses the same version of the website for every viewer (or crawler); the difference is that a script in the page code either activates the test version or not.
While both methods are valid for SEO testing, there’s a higher risk associated with client-side testing. That’s because in-page scripts may or may not interact correctly or fast enough with the search engine bots, which may result in failure to index your text versions. In addition, scripts can add to overall page load times; given that site speed is a known search engine ranking factor, it’s best to avoid using them when your specific goal is related to boosting SEO traffic.
To maximize effectiveness of your SEO test, use server-side test pages. Seek out content management and testing tools that enable server-side delivery of content, or the option of using either method, as Monetate does.
Measuring and Analyzing Test Results
Having gone through the effort of setting up test groups and implementing changes across the variant subset, you may be tempted to ease up once the experiment launches and rely on a simple performance comparison to gauge whether the variant has been successful. While this approach gives you a snapshot of which group is doing better on any given day, to account for external factors and cyclical traffic swings, you should create a traffic forecasting model.
To create a forecast, use past analytics data and seasonal traffic history to project how much traffic the control and test groups might be expected to receive during the test period. If one group exceeds or drops below the forecasted numbers, extrapolate to adjust the projection for the remainder of the test. If one group continues to outperform projections even with adjustments, you can confidently assume that difference is due to the actual page being served, rather than any external influences.
Automated test tools ease close monitoring and close adjustments. You’ll likely be able to see a dashboard of live test results and adjust forecasts in real time via the console; you may even be using a tool, like Monetate, that leverages the power of AI to recommend changes to the test as it runs. Whatever you do, stay the course and finish the test. Differences may begin showing between the two groups as soon as a week after launch, but whatever you do, run the test for its full scheduled length. Results can change over time and an adequate sample size is crucial.
Key Considerations in A/B Test Analysis
Once your test is finished, it’s time to dive into the data. You may have included multiple metrics in your hypothesis – for example, a lift in revenue or orders for eCommerce sites – but the first round of analysis should always focus on organic traffic.
That’s because the chief goal of SEO is to increase visibility with relevant audiences in search engines. Once they arrive on your site, any number of factors could influence conversion or revenue metrics – from customer service policies to inventory shortages. Organic traffic is a pure measurement of the impact of the changes you tested.
You may be tempted to layer in search engine rank tracking and click-through data, but these two data points only muddy the waters. In the current era of hyper-personalized search results, traditional rank tracking tools are almost meaningless; each user’s search results differ depending on their device, location, and prior web browsing history. While newer tools can still produce accurate ranking, there are so many factors in play that it’s impossible to assign causation to your test changes. And, of course, ranking isn’t everything; downpage links can attract more traffic, which is ultimately what leads to new business for you.
Similarly, click-through rates vary according to your links’ visibility user by user, so there are multiple variables at play. If you want to peek at click-through rates, Google’s Search Console provides a running average you can apply to your test and control groups. But they shouldn’t take precedence over organic traffic.
Measuring Impact Beyond SEO
SEO A/B split testing illuminates the otherwise seemingly-impenetrable cause and effect behind search engine visibility. While it requires resources, expertise, and plenty of traffic to implement, SEO split testing can be the first step toward a comprehensive understanding of the impact of SEO investments on business health.
Once you’ve gauged the traffic impact of test changes, you can expand your inquiry and marry that data with conversion and revenue tracking, as well as standard behavioral analytics. By tracking the full-funnel impact of SEO changes, you can determine whether you attracted relevant audiences for your offerings.
Knitting together this end-to-end process requires unification of customer profiles across touchpoints and a standardized approach to site data collection. But those efforts can unlock new insights into what site offerings are most effective and where new opportunities lie.