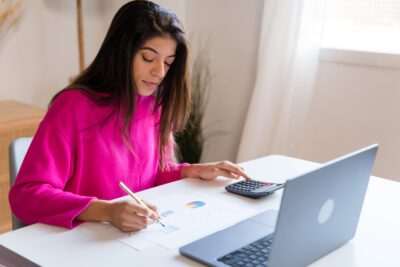
How to Calculate My A/B Testing Sample size?
Using the right A/B testing sample size ensures you’re getting reliable results from your marketing and design experiments. But there is a minimum sample size you need for every test you perform. The right sample size ensures confidence that your test results are accurate. You simply cannot draw meaningful conclusions if you don’t have enough data for a given test.
The “right” size varies on your specific needs, comfort levels, and variables like timing and budget. The goal of this post is to guide you through the process of determining the appropriate sample size for your A/B tests so you have confidence that your experiments are effective and efficient.
We’ll also explore strategies to help you manage low website traffic situations so you can still gain valuable insights from your tests. The first thing you need to understand is what we mean by “minimum sample size” and why it’s so important in A/B testing.
What is an A/B Testing Minimum Sample Size?
Minimum sample size in A/B testing is the smallest number of participants or data points required to reliably detect a difference, if one exists, between two versions (A and B) in a test. You want a sample that’s large enough to be statistically significant, but avoid wasting time and resources gathering too much data from a larger sample size than you need.
For example, let’s say you want test a purple CTA button and a pink CTA button on your home page to see which one performs better. Your sample size should be large enough to demonstrate that any observed difference in click-through rates between the two buttons isn’t random. Size depends on several things, including:
- The expected effect size – The degree of difference you anticipate
- The power of the test – The probability of detecting a real effect
- The significance level – The probability of concluding there’s an effect when there isn’t one).
If you expect a small difference between the two versions, you’ll need a larger sample size compared to expecting a larger difference. In the above example, purple and pink aren’t that different from each other, so you will need a larger sample size than if you were testing a red button versus a blue one. Thus, keep in mind that subtle differences are harder to detect and require more data.
A/B Testing Sample Size Formula
The main metrics you’ll be considering when calculating sample size include:
- Baseline conversion rate: The control group’s expected conversion rate.
- Minimum detectable effect (MDE): The minimum improvement compared to baseline that you want the experiment to detect.
Let’s say you have a website with a baseline conversion rate of 20% and you want to detect a 2% increase in conversion rate. The MDE would be 10%. To get to 10%, use the following formula:
- MDE (10%) = Desired conversion rate lift (2%) / Baseline Conversion Rate (20%) x 100%
Next, you’ll need to calculate the sample size for your test. To do that, use a sample size calculator like this one from Survey Monkey.
For math nerds, here’s the formula to calculate sample size yourself from towardscience.com:
Once you’ve figured out your sample size, you can use your website traffic to estimate how many days to run your experiment to get statistically significant results. Do that by using the following two formulas:
Total visitors needed: Sample size x total # of experiment variations = # of visitors needed
Length of experiment: Total # of visitors / avg. daily visitors = # of days to run experiment
What to do if you need more traffic to increase your A/B testing sample size?
Sometimes, you face a scenario where your traffic is very low and it’s difficult to get enough visitors to meet your minimum sample size requirements. If this is the case, here are some things you can do if you need more traffic:
Utilize your TOFU Conversions
- Focus on top-of-funnel (TOFU) conversions which tend to be higher versus those lower in the funnel. For example, instead of testing purchase completion, test newsletter sign-ups.
Use minimal variants
- Limit the number of variants in a test so you can reach conclusions faster (e.g., test two versus four version of a webpage). Apply insights from failed tests
Apply insights from failed tests
- Analyzing insights from unsuccessful tests can help you refine future ones. For example, if a previous test on a call-to-action color failed, use those insights to test different elements like button placement or font size.
Make impactful changes in tests
- Choose changes that have the potential for a significant impact when testing variants. Focus on testing major design changes, for example, versus minor copy tweaks.
Verify your variants qualitatively
- Verify your variants qualitatively by getting user feedback or expert reviews to ensure the variants are meaningful. For example, before A/B testing, conduct user interviews to gauge the potential impact of the changes to your primary navigation.
Determine the right statistical significance score
- Make sure you’re using the right statistical significance score for your business and risk tolerance. A startup might accept a 90% confidence level to move faster versus a larger, more established company that requires a 95% confidence rate.
- A/B testing is often a balancing act of getting statistically reliable results without burning through resources. It’s all about finding that right sample size for your specific scenario, one that gives you confidence in your test results without overdoing it. Luckily, solutions like Monetate take the guesswork out of this balancing act, making sure your tests hit the mark every time.
- Monetate is a personalization platform packed with business-focused A/B testing tools that simplify the process of testing and experimentation. With Monetate, you get to focus on what really matters: getting meaningful insights from your experiments, without getting bogged down in the nitty-gritty of statistics. Reach out for information on how you can use add testing to your personalization and marketing strategies with Monetate.